The last few years have witnessed rapid advancements in technologies that enable all aspects of data from data capture to business insights. Companies that don’t leverage their data assets risk ceding a key source of competitive advantage to industry rivals and can be quickly left behind in the areas of innovation, productivity, and speed to market.
A company’s capability to gain business value from its data assets is driven by its data maturity, which is built on a combination of technology, process, awareness, and adoption. A typical first step in the journey to greater data maturity is an assessment of the current state by applying a data maturity model. Data maturity models vary depending on source, but all proceed from basic or generic to transformational.
"Leadership needs to make a strategic decision to leverage its data as a strategic asset and a true market differentiator to transform into a data driven organization." |
Big Data's Promise Unrealized
According to a Gartner survey in 2018, 60% of organizations worldwide consider themselves to be in the bottom three-fifths on the scale of data maturity with, at best, a few business executives evangelizing a basic strategic vision. Users in various departments frequently find it difficult to gain insights from available data in a manner that is timely and meaningful, and as a consequence, business decisions are based on gut instinct rather than factual insights informed by data.
What little information is available is in the form of inflexible static reports delivered on a daily or weekly basis. Anything beyond that involves work requests to a “Data Services” or “Analytics” team and turnaround time could be in days or weeks. These data teams in turn endure the drudgery of constantly servicing custom data-pull requests and often suffer from low morale.
Dell Data Maturity Model
The widely used Dell Data Maturity Model categorizes organizations at four levels. According to this model, most of these companies may be classified as Data Aware.
Figure 1: Dell Data Maturity Model
Data Aware is the lowest level of this model in which an organization treats its data as merely the exhaust from its business operations. As organizations build up their data capabilities and processes, they grow through the levels of Data Proficient and Data Savvy. The ideal is to become a Data Driven organization which has mature data processes, and where nearly all business decisions are enabled by data-driven insights.
Leadership needs to make a strategic decision to leverage its data as a strategic asset and a true market differentiator to transform into a Data Driven organization. With this as a goal, companies can start by creating a data team led by a Chief Data & Analytics Officer (CDAO). In the past, companies have traditionally relegated this function to the IT department, reporting down from a CIO or CTO. As the role continues to evolve, CDAOs are increasingly reporting to the CEO, CFO or COO, sending the message that data is a critical business priority, not just an IT concern.
The new data and analytics team should begin by exploring opportunities to modernize the technology stack across the data analytics lifecycle, from data capture to data visualization.
Figure 2: The Data Analytics Life Cycle
Modern day data platforms are often built on a cloud-based architecture, which enable near limitless scalability of both compute and storage. The data platforms may be built entirely on the cloud or could adopt a hybrid approach in combination with on-premise components. A data lake for structured and unstructured data coupled with a data warehouse enables addition of new data sources and data types in an agile fashion. Advanced analytical dashboards and reports created specifically for business users (vs. data scientists) will maximize ease-of-use and self-service without the data team’s assistance or intervention.
Ensuring Data Quality
The next step is to improve the quality of the data to ensure the accuracy, immediacy, and reliability of data. High levels of data quality are achieved by implementing data governance via a combination of people, process, and technology. Best practices require creating a set of key roles including data stewards and data owners, staffed with individuals with the requisite expertise in their subject areas. Data governance processes ensure oversight of aspects such as data change management. Additionally, appropriate tools may be employed to create enabling artifacts such as a data glossary, data dictionary, and data lineage.
Maximum effectiveness may be achieved by a phased rollout of the new analytical tools, one department at a time. There are always those who prefer their legacy tools and static reports since they are familiar with it and “it just works,” even if it is not optimal. To overcome this obstacle, a company may deploy a targeted training program combined with extensive communication and socialization. This step is key to success and adoption as even the best data capabilities are not worth much if they are not widely utilized by the user community.
With increasing adoption and utilization of data driven insights, data maturity gets built into the organizational DNA and companies become capable of leveraging heretofore untapped opportunities for enhancing business value.
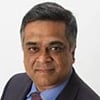
Written by Kiran Kumar
Kiran Kumar is the Chief Data and Analytics Officer at RxSense, a health-tech company providing solutions in the areas of Pharmacy Benefits Management (PBM), pharmacy discount cards, and medical and dental plans. He is responsible for all aspects of the company’s data including Data Analytics, Data Strategy, and Data Governance. Kiran has held executive level positions as CDO and CTO in the healthcare and financial services industries. His views are his own and not that of his employer, RxSense.