In this series, we have covered how to put together a data strategy by setting a data vision, how to achieve the vision, assessing the current status, setting the timeline, and change management. Now it is time to bring all these together and start implementing the data strategy.
I have broken this topic into two parts. In this article, Part 7A in the series, we will work through planning the implementation with some of the key needs and differences in a data strategy. In Part 7B we will walk through how to execute the plan by selecting the right resources and tools.
There are some key facets to a data strategy implementation that may be unfamiliar to you. Getting to know them will help you get the right resources involved to plan the milestones and resources appropriately.
Defining data scope and requirements is different from other implementations. There are some requirements such as system changes, static reports, or Key Performance Indicators (KPI) where scope and requirements are straightforward. However, since data strategies are based on integrating data with people who may not understand the current and future data, it is very difficult or impossible for them to write complete specifications on what they need. This requires some different approaches to ensure your data strategy implementation is successful.
Data Discovery or Proof of Concept
During the assessment your team looked at the Master Data, Metadata and transactional data at a high level, along with some initial data quality checks. While this was critical for the assessment it was not at the level of detail needed for implementing the data strategy. Data is like many other parts of life – you can read about it, talk about it and write about it, but experiencing it (putting your hands on it) to see how it supports your operations and analytics is invaluable. Therefore, data discovery sessions are critical for understanding data quality, defining or changing attributes, hierarchies, granularity and temporal or time variant needs. They can also be helpful in understanding what the correct system of record is for the data at what each part of your process. It is important for the data architects, data owners, data security and key users to be part of this process.
In planning for the data discovery, think through the complexity of the data you are integrating and the knowledge of the users. Some data scenarios my need multiple rounds. However, the big benefit is that your specifications are much tighter since you already have proven out the data and your development should be faster with significantly reduced need for any rework.
Data Discovery = more effort up front with better specifications, adoption and less rework.
Data Alignment and a Single Version of the Truth
One of the most important elements in a data strategy is trust. The users need to trust that the data is what they expect. This is done through aligning the data definitions for every data element across the organization. While this may seem to be a herculean effort, much of the data already has definitions from the systems where they are created. However, as soon as data crosses systems or even modules within large systems there is an effort to revalidate.
Data alignment should get started as soon as possible. In fact, building a team coming out of the assessment process can be very beneficial.
Data Alignment = common language that removes assumptions and conflicts in data usage.
Other articles in this series, by Rich Peters: 1.Delivering Value Through Data 2. How to Write Your Data Vision and Mission Statements 3. How to Build a Data Strategy - Part 1: Key objectives and capabilities 4. How to Build a Data Strategy - Part 2: The assessment 5. How to Build a Data Strategy - Part 3: The roadmap |
Balancing Data Access and Data Security Through the Use of Roles
For some data, having tight controls on access is important, such as personal or internal financial data. However, the great value in data is when you combine it to tell the full story, and then let people access it. There is a balance between access to data and securing it. The data discovery process can help show benefits and risks as the data is combined. The key players in this part of the implementation are the data owners who determine the benefits and risk, the data architects who structure the data in a way to meet needs, and the data security owner. System architects may also be needed if the system needs to be configured differently to meet the needs.
One way to approach data access is to look at the data a person needs for his or her specific role, and then evaluate related data that are upstream or downstream in the process to look at the risks and benefits. Many times, it is access to the related data that solves complex issues. Many people are inquisitive by nature and letting them see data that are related to their role, even if tangentially, can reap large benefits. Constraining access to data reduces the value of the data, shows a mistrust of the employees, and reduces morale and job satisfaction.
Data Access & Security = define a consistent approach to drive value and minimize risk
Data Governance and Data Policies
Data governance forms your interactions with the data – i.e., who owns it, how it is managed, how it is secured and the data quality itself. When you are looking at the scope of effort for the implementation it is very important that you allow for the time to ensure that data governance needs are met prior to kicking off delivering each strategy element. For instance, you don’t need to define the ownership, management, security or quality for all data elements prior to starting your data strategy implementation. What is critical is that you put the governance in place prior to implementing each strategy element.
Data Governance: ensure it is set up prior to starting each data strategy element.
The hierarchy of analytics with metrics and Key Performance Indicators (KPI)
Analytics, metrics and KPI’s are a key part of any data strategy. Sometimes they are broken out as stand-alone deliverables, while at other times they are integrated into other elements. Ensuring that you have a consistent approach on how your analytics, metrics and KPIs are delivered incorporates many of the facets that are mentioned above. Data discovery will ensure you that find the right data to use; data alignment will ensure that the data are defined and used correctly; data access will ensure that the right people will have access to it; and data governance will ensure it is accurate. Your KPI’s are the top level in your information hierarchy. This means that all metrics and analytics that feed into the KPI must be defined regardless of where the raw data come from. You may have hidden dependencies in your data that will be uncovered during the implementation. Building the program team with the right process and data knowledge can mitigate these issues.
KPI Hierarchy: Look at your metrics and analytics to find data relationships and ensure consistency across the organization
Getting Started
The first step in implementing your data strategy is putting together your core implementation planning team. The minimum core team consists of your data strategy leader, a project manager with data knowledge and experience and a data architect. Some organizations may want the team to be slightly larger depending on the size and complexity of the implementation. The core team uses the deliverables outlined in the roadmap and performs detailed planning for the deliverables in the near-term (12-24 months) and more granular planning than the assessment focused on key resources, milestones, dependencies and budget for those deliverables that are more than 24 months in the future. The core team will leverage subject matter experts while building out the plan to ensure critical knowledge is incorporated into the planning.
Objectives of initial implementation planning:
Milestones and dependencies (leveraging the roadmap)
- All defined deliverables with dependencies (especially the data and people dependencies)
- Proof of Concept (POC) for tools and process
- Data discovery or data “POC”
- Ensuring resources are available for the early “quick wins”
- Alignment or conflict with all other initiatives or constraints for the organization
Defining resources (helps define extended planning team)
- Key business or process resources
- Key IT resources
- Key data resources
- Restraints due to ongoing operations or other initiatives
- Do you need external resources for tool implementation, data best practices and change management? If so, allow time for knowledge transfer throughout the planning.
Change management and educating the users
- Early evaluation of change management effort
- Identifying leadership as evangelists and champions
Options based on organizational priorities, investments, constraints and POC outcomes
- Early previews to leadership to show investments and resource commitments
- Contingency planning for POC outcomes
- Documenting risk based on other commitments or constraints the organization faces
- Detailed dependencies showing what elements can be shifted easily and those that by nature are more sequential
- Documented foundational costs that enable later deliveries and ensure these are well understood. These go to overall program costs and ROI, but may not be easy to allocate to later deliverables. Examples include data management efforts, infrastructure and tool costs.
- Refining and documenting direct costs and ROI for individual elements on the roadmap that are not foundational.
Deeper assessment of elements on roadmap to ensure accurate and cost-effective delivery
- Deeper dive by SME’s to catch any gaps or inconsistencies
- Looking for ways to gain synergies across elements
- Ensuring that data foundational efforts such as data alignment and data quality and governance are aligned with other deliverables
Finalizing the plan
Once the initial planning is done, it is time to take it to the extended team for adjustments and commitments. The extended team consists of the following people; Organization leadership, IT leadership, Process leadership, Change Management and Enterprise architecture. Each of these groups may bring in subject matter experts (“SMEs”) to flesh out or validate details. Resource gaps will be addressed in part 7B of this series.
- Does the proposed plan still deliver the value expected as identified in the roadmap? During planning, hidden dependencies or other constraints may have shifted the sequence of deliverables.
- Does each internal team have the resources needed to deliver on their commitment? Specific names should be assigned based on the knowledge and experience they bring to the team.
- Can the organization absorb both the cost and the change required for a successful implementation? This is when the reality of the investment and change come to light. It is critical to get a full buy-in to the plan or shift the plan to meet the cost or change needs.
- Are there other organizational constraints that can impact the implementation? The leadership needs to bring forward any other organizational commitments or risks that could impact the implementation and be clear on where this implementation fits in order of priority. Preplanning for when or if resources could be pulled for other issues is critical for the overall success.
- Are there any assumptions that are no longer valid or have higher risk? All plans are made with assumptions. Being explicit and having options for changes is necessary.
- Are the proof of concept elements well defined with options if they do not pan out?
- Are the critical components and the critical path well defined for each end state deliverable? Be very focused on the data and metadata aspects of the implementation. Many times, tools and processes are faster to implement than the data and metadata. This is due to getting consensus from all the stakeholders.
- Is the sizing and costing of each of the elements consistent and reasonable? Do each of the teams understand the time and resource commitment along with the deliverables? Is the time commitment consistent with the expected effort? This is usually not an issue if the teams were consulted and integrated into the core planning process.
- Are all the deliverables encompassed in the planning? For some large implementations only the first phase of deliverables is planned at the detail level. It is best practice to get a better estimate for the subsequent phases so there is a better understanding of the overall cost and resource commitments. That said, it is not a full detailed plan, but one that uses the knowledge from the initial planning to give better estimates that the leadership can use in evaluating investments.
In the next article in this series we will shift from planning the implementation to securing the resources, selecting the tools, and executing the plan.
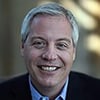
Written by Rich Peters
Rich Peters is a seasoned executive with 25+ years of data strategy, business intelligence, and solutions implementation experience across various industries. He is the Founder of Tongere Partners, which helps companies become more successful through the alignment of their data with their business strategy and processes.